Social Impact Assessment in Innovation Projects via STATA, Methods:: Causal Inference, Randomization, Propensity Score Matching, and Double-Difference
Keywords:
social impact, innovation, STATA, double-difference, propensity score matching, randomization, causal inferenceSynopsis
In the business and industrial world, the design and implementation of projects typically take into account the economic, financial, and even political or environmental impact when introducing innovations. However, in the first two decades of the 21st century, various events have demonstrated that the evaluation of social impact on well-being generation is of vital importance.
In fact, in the times of the new normality envisioned as the Post-COVID-19 era, this becomes particularly relevant since all policies and actions undertaken by companies and governments must have the necessary endorsement of a social impact evaluation when introducing innovations.
For this reason, the work: "SOCIAL IMPACT EVALUATION IN INNOVATION PROJECTS VIA STATA. Methods: Causal Inference, Randomization, Propensity Score Matching, and Double Difference," Volume 1 is aimed at describing both to those familiar and unfamiliar with the topic, what social impact is, its characteristics, conditions, and implications, the main methods used to calculate it as well as the opportunities that arise in the Post-COVID-19 era, which demands that innovation resources and actions designed and implemented reflect high standards of social impact that foster well-being, particularly in emerging countries.
To achieve this, this work is divided into a collection of two volumes, corresponding to Volume I:
Chapter 1. Basic Concepts. This chapter is aimed at explaining what social impacts are, the reasons for evaluating them, the differentiation between monitoring vs. evaluation, the indicators of both, what a results-based monitoring entails, and its configuration. This allows for a better definition of the idea of impact evaluations, their relationship as studies of efficacy and effectiveness, the implications of operational evaluation as well as their qualitative and quantitative impact characteristics, in addition to the reference point where impact evaluation is required, either prospectively or retrospectively. Conducting any social impact evaluation for the introduction of innovations requires recognizing the treatment and control groups, which necessitates solving the problem of determining the counterfactual groups; with and without comparisons as well as their relationship with bias. A case description is provided, explaining selection bias in an ex-post scenario and explaining the characteristics that accompany it, such as process evaluations and project evaluations, the importance of cost-benefit and cost-effectiveness analysis as well as ethics in social impact evaluation in the introduction of innovations.
Chapter 2. Preparation, causal inference, and counterfactuals.
Given the preparation of treatment and control groups, this chapter is designed to consider the steps that serve the construction of counterfactual scenarios that enable groups to explain the construction of a theory of change, explain the links in a results chain, the techniques that facilitate the specification of evaluation questions, the selection of outcome and performance indicators, and to create a data-based checklist for the indicators. This equips the researcher to perform causal inference, describe and estimate counterfactuals, through two case studies with which the chapter concludes.
Chapter 3. Randomization. It is from this chapter until chapter 7, where different techniques based on STATA 14 software are introduced, so it is recommended, if not familiar with the software, to start with the Appendix: Introduction to STATA. This will allow you to understand the operating rules as well as to access the example case files that will be discussed and that are hosted at the link: [link]. Thus, Chapter 3 will allow the reader to understand the characteristics of random assignment, advantages and disadvantages of its use, the recommended conditions for its application, the ethical implications, the different randomization methods that exist, checklists to carry it out as well as an example with STATA for understanding its use.
Chapter 4. Propensity Score Matching (PSM). It explains to the more avid reader, what this technique is and does, the assumptions and rules that must be met for its application, steps to achieve it as well as the use of complementary techniques. Its advantages and disadvantages of use are debated and it is completed with an example in STATA to check its understanding of use.
Chapter 5. Double Difference (DD). The chapter is designed to explain the method, what it is, how it is used, what happens in it through the use of fixed effects panel, how to implement it, advantages and disadvantages, its usefulness, trends, alternative models, the possibility of combining it with PSM, adjustments, limitations, concluding with an example in STATA to check its understanding of use.
Downloads
References
Abadie, A. J.; Imbens, G. (2008). On the failure of the Bootstrap for Matching Estimators. Econometrica 7 (6): 1537-1557
Abadie, A. J.; Angrist, D.; Imbens, G. W. (2002). “Instrumental Variables Estimates of the Effect of Subsidized Training on the Quantiles of Trainee Earnings. Econometrica 70 (1): 91–117.
AIEI (2015). Evaluación de Impacto Social: Lineamientos para la evaluación y gestión de impactos sociales de poryectos. Asocia- ción Internacional para la Evaluación de Impactos. Banco Intera- mericano de desarrollo, en: https://www.iaia.org/pdf/Evaluacion- Impacto-Social-Lineamientos.pdf
Bamberger, M.; Rao, V. y Woolcock, M. (2010). Using Mixed Methods in Monitoring and Evaluation: Experiences from International Devel- opment. Documento de trabajo de investigación de políticas Núm. 5245. Washington, D.C.: BancoMundial.
Becker, S. O. e Ichino (A). (2002a). Stata programs for ATT estimation based on propensity score matching, en: http://sobecker.userweb. mwn.de/pscore.html
Becker, S. O. e Ichino, A. (2002b). Estimation of Average Treatment Effects Based on Propensity Scores. Stata Journal 2 (4): 358–77.
Bell, B.; Blundell, R. y Van Reenen, J.(1999). Getting the Unemployed Back to Work: An Evaluation of the New Deal Proposals. Interna- tional Tax and Public Finance 6 (3): 339–60.
Bernal, R. y Peña X. (2011). Guía práctica para la evaluación de impacto. Perú: Universidad de los Andes: Facultad de Economía.
Bertrand, M.; Dufl E. y Mullainathan, S. (2004). How Much Should We Trust Differences-in-Differences Estimates? Quarterly Journal of Economics 119 (1): 249–75.
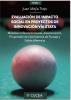
Downloads
Published
Series
Categories
License

This work is licensed under a Creative Commons Attribution-NonCommercial 4.0 International License.