Multivariate Statistics. VOLUME I. Dependent Techniques with SPSS in the Social Sciences
Keywords:
multivariate statistics, dependent techniques, spss, social sciencesSynopsis
Multivariate analysis is present in various software applications, thereby increasing its usability across different disciplines such as Administrative Sciences. Some of the most widely used software in both academic and professional fields worldwide include the Statistical Package for the Social Sciences (SPSS, by IBM), Analytics, Business Intelligence and Data Management (SAS, by SAS Institute and/or World Programming), Statistica (by STATISTICA), and the R language (open-source software). Given this, it is not surprising that Administrative Sciences support academic development through various postgraduate programs and in the professional world related to Social Sciences. Consequently, there is a growing trend in the publication of reports, articles, book chapters, or books discussing various theoretical and empirical aspects and their interpretation based on these software applications. In our case, we adopt IBM SPSS 20 for the development of the topics in this book.
Based on the above, we present this work with a triple purpose:
- To present a document that serves both those familiar and unfamiliar with the topic, who need to understand both the concepts addressed in this volume and how to manipulate the various commands offered by IBM SPSS 20 regarding the example problem cases presented.
- For a better understanding of case treatments, we follow the sequence proposed by Hair et al. (1999) of the six steps: objectives, design, assumptions, execution, interpretation, and validation, as the axis for presenting and resolving these cases.
- As the Coordinator of the Doctorate in Administrative Sciences at the University Center for Economic and Administrative Sciences (CUCEA) of the University of Guadalajara (UdG), to present the foundational book for the Quantitative Methods I and II courses.
The author hopes to contribute to the reader's acquisition of knowledge that can be applied in the practical world and aid in its theoretical interpretation. If this is not the case, it is hoped that at least it will serve as another useful step in achieving their academic and/or professional development.
Downloads
References
Addelman, S. ( 1962), Orthogonal Main-Effects Plans for Asymmetrical Factorial Experiments. Techno metrics 4: 21-46.
Akaah, l. ( 1991 ), Predictive Performance of Self- Explicatcd, Traditional Conjoint, and Hybrid Conjoint Modcls undcr Alternative Data Collection Modcs. Journal of the Academy of Marketing Science 19:309-14.
Allenby, G. M., Arora, N., y Ginter, J. L. (1995), Incorporating Prior Knowledge into the Analysis of Conjoint Studies. Journal of Marketing Research 32 (May): 152-62.
Alpert, M. ( 1971), Definition of Determinan! Attributes: A Comparison of Methods. Journal of Marketing Research 8(2): 184-91.
Baalbaki, I. B., y Malhotra, N. K. (1995), Standardization versus Customization in Intemational Marketing: An Investigation Using Bridging Conjoint Analysis. Journal of the Academy of Marketing Science 23(3): 182-94.
Bretton-Clark (1988), Conjoint Analyzer. New York: Bretton-Clark. Carmone, F. J., Jr., y Schaffer, C. M. (1995),Review of Conjoint Software.
Journal of Marketing Research 32 (February): 113-20.
Carroll, J. D., y Green, P. E. (1995), Psychometric Methods in Marketing Research:
Part 1, Conjoint Analysis. Journal of Marketing Research 32 (November): 385-91. Conner, W. S., y Zelen, M. (1959), Fractional Factorial Experimental Designs for
Factors at Three Levels, Applied Math Series S4. Washington, D.C.: National Bureau of
Standards.
Elrod, T., Louviere, J. J. y Davey, K. S. (1992), An Empírical Comparison of Ratings-
Based and Choice- Based Conjoint Models. Journal of Marketing Research 29: 368-
Green, P. E. (1984), Hybrid Models for Conjoint Analysis: An Exploratory
Review. Journal of Marketing Research 21 (May): 155-69.
Green, P. E., Goldberg, S. M., y Montemayor, M. (1981), A Hybrid Utility Estimation
Model for Conjoint Analysis. Journal of Marketing 45 (Winter):33-41.
Green, P. E., Kreiger, A. M., y Agarwal M. K. (1991), Adaptive Conjoint Analysis: Sorne
Caveats and Suggestions. Journal of Marketing Research 28 (May): 215-22. Hahn, G. J., y Shapiro S. S. (1966), A Catalog and Computer Program for the Design
Huber, J. (1987), Conjoint Analysis: How We Got Here and Where We Are, In
and Analysis of Orthogonal Symmetric and Asymmetric Fractional Factorial Experiments, Report No. 66-C-165. Schenectady, N.Y.: General Electric Research and Development Center.
Hair , J.F.; Anderson, R.E.; Tatham, R.L.; Black W.C. .Análisis Multivariante.1a. Ed. Espana. Prentice (all.
Proceedings of the Sawtooth Conference on Perceptual Mapping, Conjoint Analysis and Computer lnterviewing, M. Metegrano, cd., Ketchum, Idaho: Sawtooth Software, pp. 2-6.
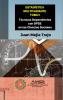
Downloads
Published
Series
Categories
License

This work is licensed under a Creative Commons Attribution-NonCommercial 4.0 International License.