Qualitative Comparative Analysis. VOLUME II. FUZZY-SET (fsQCA) Theory and Practice
Keywords:
qualitative comparative analysis, fuzzy-set, fsQCA, theory and practiceSynopsis
The present document is a continuation of the work, Crisp-Set Qualitative Comparative Analysis (csQCA) and its Relationship with Innovation: Discussion and Interpretation of Results, and aims to introduce researchers to the concepts and applications involved in the case of Qualitative Comparative Analysis (QCA) with fuzzy-set data (fsQCA) through demonstrations of its use with various examples in the administration of innovation.
Since the late 1980s, its precursor, Qualitative Comparative Analysis (QCA), has been at the forefront of social science methodology as it is based on the set of relationships and established objectives for discovering sufficient and necessary conditions.
It is worth noting that it is common to refer to the original Boolean version of QCA as csQCA, where cs (crisp-sets) denotes a set of crisp data. The version that allows for the use of multiple-category conditions is called mvQCA, where mv (multi-value) describes the multi-value aspect, and fsQCA where fs (fuzzy-set) describes the fuzzy-set data. The goal of QCA analysis, in general, is to account for a particular outcome, unlike regression-based analyses, which typically aim to be fundamental tools for explaining the effects of causes (Wagemann and Schneider, 2010).
Regarding the state of social sciences, as a general basis for the administration of innovation, Sartori (1970) stated:
"...the science is in a poor state, oscillating between two weak extremes: unconscious thought, which is predominant, and overly conscious thought, done by a small minority..."
Calling on scholars to acquire training in basic logic:
"To guide an intermediate course between crude logical mishandling on one side and logical perfectionism (and paralysis) on the other side..."
It was in the late 1980s that Dr. Charles Ragin brought Boolean algebra and set theory to social sciences with his innovative book, The Comparative Method (Ragin, 1987), which deeply describes all components of QCA. Even so, real attention began to surge some years later with his book Fuzzy-Sets Social Science (Ragin, 2000). By now, there are scholars who use configurational comparative methods, given the possibility of formalizing case-oriented analysis, thus providing tools to enhance comparative research. These methods are particularly apt for identifying the minimally necessary and/or minimally sufficient (combinations of) conditions that produce an outcome of interest (i.e., evaluating the causes of effects), with great potential for quantitative applications in fields like engineering (Mendel and Korjani, 2010; Marks, et al., 2018).
Given the advantages offered, recent discussions on configurational comparative methods suggest that regression-based quantitative approaches and QCA are best applied alongside each other (Ragin, 2008; Schneider and Wagemann, 2010; Rihoux, 2006). However, there is a warning for enthusiasts that scholars should not become QCA monomaniacs (Ragin and Rihoux, 2004, p. 6).
On the other hand, initial efforts of applying fsQCA in entrepreneurship and innovation, such as the work by Kraus et al. (2017), compile 77 articles published from 2005 to 2016 with keywords: fsQCA, business administration, entrepreneurship, and innovation, revealing a gradual increase in these fields for using fsQCA. Thus, administrative sciences oriented towards innovation can leverage the advancements in social sciences through Dr. Ragin's significant contributions. Consequently, this work comprises eleven chapters, which we briefly describe:
CHAPTER 4. Qualitative Comparative Analysis of Fuzzy-Set Data (fsQCA). This chapter introduces the reader to a very special type of data, the fuzzy type used by fsQCA software, revealing basic concepts through understanding its nature and the possibility of using them at levels and continuously. It presents fuzzy-set data operations such as Boolean negation, conjunction (logical AND), and union (logical OR). It includes models and calibration methods, analysis of necessary and sufficient conditions and consistency, how to create truth tables, and analyze vector space corners. Fourteen exercises demonstrate the use of the fsQCA software, confirming the main concepts.
CHAPTER 5. Evaluation of the fsQCA Data Set. This chapter describes the importance of what are known as INUS and SUIN conditions as well as consistency and coverage in general. It presents the manual development of five cases that confirm the main concepts.
CHAPTER 6. Calibration and Its Importance in fsQCA. This chapter opens a debate on the implications of calibration before starting measurements in scope and context, the pros and cons of using indicators by quantitative research, SEM vs. fsQCA relationship, qualitative research, and the need for calibration, direct and indirect calibration methods. The manual development of four cases confirms the main concepts.
CHAPTER 7. Configurational Thinking in fsQCA. This chapter demonstrates the importance of configurational thinking from both qualitative and quantitative perspectives, discussing the evaluation of the degree of membership in a configuration and comparing causal pathways.
CHAPTER 8. Net Effects in fsQCA. This chapter describes what is meant by net effects, the associated problems, a proposed shift to case types, comparison of fuzzy data cases, and configuration analysis.
CHAPTER 9. Net Effects vs. Configurations in fsQCA. Through two hypothetical cases, the study develops each of the concepts seen, explaining by comparison their results, to understand the nature of net effects versus configuration effects by fsQCA.
CHAPTER 10. csQCA Analysis Guide. With crisp data from an innovation case, this chapter offers the reader a quick and visual guide through screenshots and eight exercises on how to solve a case through necessary condition analysis, representing data in the truth table, identifying contradictory and logical remainders, sufficiency analysis, truth table minimization for occurrence and non-occurrence of the phenomenon, and result interpretation.
CHAPTER 11. fsQCA Analysis Guide. With fuzzy data from an innovation case, this chapter offers the reader a quick and visual guide through screenshots and nine exercises on how to solve a case through accessing the work file, necessary condition analysis with phenomenon occurrence and reporting, interpreting coverage, generating the truth table, applying the Standard Analyses option, interpreting generated solutions, generating the truth table with phenomenon non-occurrence, analyzing main implications, and interpreting the case.
GLOSSARY. A glossary of the most commonly used terms in this context is provided.
Downloads
References
Achen, Ch. (2005). Let’s Put Garbage-Can Regressions and Garbage-Can Probits Where They Belong. Conflict Management and Peace Science. 22: 327–39.
Allison, P. D. (1977). Testing for Interaction in Multiple Regression. American Journal of Sociology 82, pp. 144–53.
Amenta, E. y Poulsen, J. D. (1996). Social Politics in Context: The Institutional Politics Theory and Social Spending at the End of the New Deal. Social Forces 75, pp. 33–60.
Bandura R. (2006). A Survey of Composite Indices Measuring Country Perfor- mance: 2006 Update, United Nations Development Programme – Office of Development Studies, en: https://www.undp.org/content/dam/undp/ library/corporate/Development%20Studies/measuring_country_perfor- mance_2006update.pdf
Bendix, R y Beger, B. (1959). Images of Societies and Problems of Concept Formation in Soiology, en Gross I. (Eds.). Symposium on Sociological Theory. NY: Harper and Row.
Bol, D. y Luppi, F. (2013). Confronting Theories Based on Necesary Relations. Making the Best of QCA Possibilities. Political Research Quarterly 66(1), pp. 205-210.
Bollen, K. A. (1989). Structural Equations with Latent Variables. New York: Wiley Interscience.
Boswell, T. y Brown, C. (1999). The Scope of General Theory. Methods for Linking Deductive and Inductive Comparative History. Sociological Methods and Research 28, pp. 154–85.
Braumoeller, B. y Goertz, G. (2000). The Methodology of Necessary Condi- tions. American Journal of Political Science 44, pp. 844–58.
Byrne, D. (2002). Interpreting Quantitative Data. Londres: Sage.
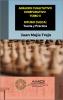
Downloads
Published
Series
Categories
License

This work is licensed under a Creative Commons Attribution-NonCommercial 4.0 International License.