Social Impact Evaluation. VOLUME I. Use of STATA with Methods: Causal Inference, Randomization, Propensity Score Matching, and Double-Difference
Keywords:
social impact evaluation, STATA, causal inference, randomization, propensity score matching, double-differenceSynopsis
In the business and industrial world, the design and implementation of projects usually consider economic, financial, and even political or environmental impacts when introducing innovations. However, in the first twenty years of the 21st century, various events have demonstrated that the evaluation of social impact in generating well-being is of vital importance.
In fact, in the times of the new normal expected in the post-COVID-19 era, this becomes particularly relevant since all policies and actions issued by companies and governments must have the necessary approval of a social impact evaluation for the introduction of innovations.
For this reason, this work is aimed at describing to both insiders and outsiders what social impact is, its characteristics, conditions, and implications, the main methods used to calculate it, as well as the opportunities that loom in the post-COVID-19 era, which demands that the resources and actions of innovation design and implementation reflect high standards of social impact that promote well-being, particularly in emerging countries.
To achieve this, this work is divided into a collection of two volumes, with Volume I covering:
Chapter 1. Basic Concepts. This chapter is aimed at explaining what social impacts are, the reasons for evaluating them, the differentiation between monitoring vs. evaluation, the indicators of both, what result-based monitoring implies, and its configuration. This allows for a better definition of what impact evaluations are, their relationship as efficacy and effectiveness studies, the implications of operational evaluation as well as their qualitative and quantitative impact characteristics, and the reference point at which the impact evaluation is required, whether prospective or retrospective. Conducting any social impact evaluation for the introduction of innovations requires recognizing the treatment and control groups, which needs to solve the problem of how to determine the counterfactual group; with and without comparisons, as well as its relationship with bias. A case description is provided, explaining selection bias in an ex-post scenario and detailing the accompanying characteristics such as process and project evaluations, the importance of cost-benefit and cost-effectiveness analysis, and the ethics in social impact evaluation in the introduction of innovations.
Chapter 2. Preparation, Causal Inference, and Counterfactuals. Given the preparation of the treatment and control groups, this chapter is designed to consider the steps that serve to construct counterfactual scenarios that enable the groups to explain the construction of a theory of change, the links in a results chain, the techniques that facilitate the specification of evaluation questions, the selection of outcome and performance indicators, and creating a data-based checklist for the indicators. This equips the researcher to conduct causal inference, describe, and estimate counterfactuals, through two case studies that close the chapter.
Chapter 3. Randomization. From this chapter through Chapter 7, different techniques based on STATA 14 software are introduced, so it is recommended to start with the Appendix: Introduction to STATA if you are not familiar with the software. Chapter 3 will allow the reader to understand the characteristics of random assignment, the advantages and disadvantages of its use, the recommended conditions for its application, ethical implications, the different randomization methods that exist, checklists for carrying it out, and an example with STATA for comprehension.
Chapter 4. Propensity Score Matching (PSM). This chapter explains to the more diligent reader what this technique is and does, the assumptions and rules that must be met for its application, steps to achieve it, as well as the use of complementary techniques. Its advantages and disadvantages are debated, and it is completed with a STATA example to verify its comprehension and use.
Chapter 5. Difference-in-Differences (DiD). This chapter is designed to explain what the method is, how it is used, what happens in it through the use of a fixed effects panel, how to implement it, advantages and disadvantages, its utility, trends, alternative models, the possibility of combining it with PSM, adjustments, limitations, concluding with a STATA example to verify its comprehension and use.
Downloads
References
Abadie, A. J.; Imbens, G. (2008). On the failure of the Bootstrap for Matching Estimators. Econometrica 7 (6): 1537-1557
Abadie, A. J.; Angrist, D.; Imbens, G. W. (2002). “Instrumental Variables Estimates of the Effect of Subsidized Training on the Quantiles of Trainee Earnings. Econometrica 70 (1): 91–117.
AIEI (2015). Evaluación de Impacto Social: Lineamientos para la evaluación y gestión de impactos sociales de poryectos. Asocia- ción Internacional para la Evaluación de Impactos. Banco Intera- mericano de desarrollo, en: https://www.iaia.org/pdf/Evaluacion- Impacto-Social-Lineamientos.pdf
Bamberger, M.; Rao, V. y Woolcock, M. (2010). Using Mixed Methods in Monitoring and Evaluation: Experiences from International Devel- opment. Documento de trabajo de investigación de políticas Núm. 5245. Washington, D.C.: BancoMundial.
Becker, S. O. e Ichino (A). (2002a). Stata programs for ATT estimation based on propensity score matching, en: http://sobecker.userweb. mwn.de/pscore.html
Becker, S. O. e Ichino, A. (2002b). Estimation of Average Treatment Effects Based on Propensity Scores. Stata Journal 2 (4): 358–77.
Bell, B.; Blundell, R. y Van Reenen, J.(1999). Getting the Unemployed Back to Work: An Evaluation of the New Deal Proposals. Interna- tional Tax and Public Finance 6 (3): 339–60.
Bernal, R. y Peña X. (2011). Guía práctica para la evaluación de impacto. Perú: Universidad de los Andes: Facultad de Economía.
Bertrand, M.; Dufl E. y Mullainathan, S. (2004). How Much Should We Trust Differences-in-Differences Estimates? Quarterly Journal of Economics 119 (1): 249–75.
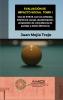
Downloads
Published
Series
Categories
License

This work is licensed under a Creative Commons Attribution-NonCommercial 4.0 International License.